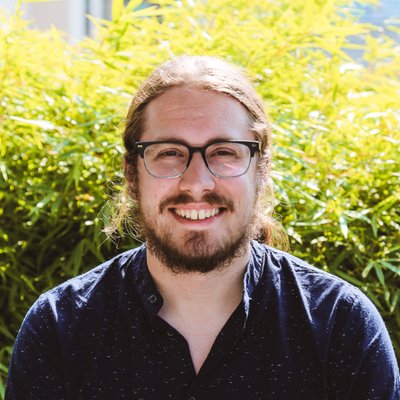
Senior Developer Advocate for Generative AI at Amazon Web Services
Dec 1, 2022 44 min watch
Build your application easily and efficiently with serverless containers
I delivered this talk at AWS re:Invent 2022, as part of the serverless containers track. It compares the ease and efficiency of AWS Lambda, AWS App Runner, and AWS Fargate, with a particular focus on the built-in features of each compute model, and how it scales as you receive large amounts of concurrent web traffic.
You can watch the recording, download the deck, and read the transcript with slides below.
Transcript
Today I’m going to be talking about how to build your application easily and efficiently with serverless containers. “Easy” and “efficient” are definitely topics that I love to talk about, and “serverless containers” are another area that I work in. I work as a developer advocate for container services at AWS.
So to kick things off, I want to introduce an idea that I’ve developed over years of software development at different startups, and that is that as a software builder, you need the right tools at the right time. So similar to growing plants: if I was to use a watering can on a full grown tree, it’s not going to do much for that tree. Likewise, if I use a rake on a tiny baby plant, it’s probably just going to kill that plant. I need to use the right tools for developing that plant, in it’s life cycle.
The same thing goes for software. If I’m building something that’s completely new in the software space, the main thing I generally need is rapid product development because I need to build new features. I need to figure out what fits with my intended market and how to build what they need in order for that product to be a success. But if I’m building on a piece of established software that already has a successful established user base, maybe what I need more is support, maintenance, reliability, and maybe feature development slows down a little bit. But I have different needs as a software builder.
So when looking at ease and efficiency, the two dimensions that we’re going to talk about in this talk, the main thing to look at is finding the right spot on these two dimensions for where your software and where your development cycle currently is.
When I look at ease and efficiency, the questions I ask myself are:
- Does this tool help me build better and faster right now?
- Or have I outgrown this tool and it’s now starting to get in my way of what I’m trying to build in terms of efficiency?
- Is this tool’s pricing, computational model, or its optimization appropriate for where I am right now in terms of scaling?
- Have I outgrown this tool, or is it fitting what my needs are in terms of pricing model?
I believe that containers are a tool that helps with both of these dimensions, ease and efficiency, all the way from a brand new greenfield project up to an established software that’s been around for decades.
I’m going to show those two dimensions, first for greenfield projects. What I’ve found with containers is that for a greenfield project, a container is going to get you started a lot faster. There are literally millions of prebuilt container images on Docker Hub, and many of those are production ready. They’re patched. They’re maintained by core teams that develop runtimes themselves, like the Python core team or Node.js core team. So they’ve built a production ready pattern for distributing a particular runtime for your application. And all I have to do as a developer is pull that off Docker Hub and start developing on top of it. That speeds up my development on a greenfield project.
Additionally, building your own deploy tooling isn’t the greatest use of your time if you’re trying to build a business, and likewise goes for local development. If I’m spending a lot of time building out a deploy chain, a build chain, and a chain for shipping my application to production, that’s all wasted time that could have been spent on building business features to deliver value to my end customers faster.
Now, meanwhile, on the other end of the spectrum with an established software, what I found is that containers help solve a problem of standardization. Often, especially as a company grows, you end up with a lot of different competing tooling, competing standards, and competing formats for how to build an application and ship it to production. Docker and Docker containers provide a standard format that not only eliminates these special snowflakes where the deployment tooling is only known by a couple of people inside the company, but it also means that you can bring in an employee from anywhere that uses Docker containers and they’re going to be more or less familiar with the same tool chain.
Additionally, for established businesses, you’ll find that containers help you pack applications on infrastructure more efficiently to save money. You’re not going to have as many VM’s that are sitting there running at extremely low utilization but still costing a lot of money.
And there’s reliable deployments and rollbacks. A container image, once it’s deployed, is immutable. And even if I have something that happens terrible in that environment where my application crashes, it goes down and completely destroys the local file system, I can always bring that container image back onto that machine, reboot it from scratch and restore it based on the pattern that’s supposed to be running in.
Now, I’ve talked a little bit about ease and efficiency, but I want to talk about efficiency more because this really goes into the cost that you’ll face when you’re developing applications. And concurrency is at the root of all efficiency problems. Computers, throughout their development, have enabled us to do more things, more quickly, with more concurrency.
I want to talk about a little bit of the timeline of that, starting with the Tandy 1000, which was the first computer that I actually wrote code on. I loved this computer, but it had an extremely low power processor: 4 MHz. It was only really capable of running one program at a time and in fact, if you wanted to run another program, you had to physically turn the machine off, take the disk out, put a new disk in it and turn it back on. So no concurrency really going on there.
Now, fast forward a few years. We get Windows 3.1, we get cooperative multitasking and the idea that you could have multiple programs running at the same time on a slightly more powerful processor, but they had to coordinate periodically. Each program was responsible for checking with the operating system to see if another background program wanted to use the CPU. And if there was, then that program could choose to yield its time to that other program in the background. It required every program to be implemented in this manner and to implement cooperative multitasking properly. So it didn’t always work. A lot of times you would have programs that would freeze up the entire computer.
So processors continue to get more powerful. A few years later, we get Windows 95, Windows 98. We’re starting to get preemptive multitasking. In this approach, the operating system is actually watching over that CPU and it is freezing programs and switching out which program is running on the CPU core on the fly. Programs no longer have to do cooperative multitasking. Instead, the operating system is taking over and saying: “Get off the processor. It’s time for another program to actually run on the processor right now.” It’s switching back and forth really quickly to give the illusion of multitasking.
A few years later, we get multicore processors. For the first time, we actually have processors which have two cores that can run two independent programs at the exact same time in parallel with each other which led to a whole new level of innovation and speed and concurrency in applications.
Where we are today is client/server architecture. A lot of the programs that we use day to day, they don’t just run locally on your computer, they communicate over the Internet. And one interaction that you have on your local client could kick off tens or even hundreds of servers in the background that are coordinating to answer your request and make something happen in response to your click or your interaction in this application.
And when we talk about concurrency, these servers are often handling concurrent requests requests from tens, hundreds, thousands, maybe even millions of different connected clients at the exact same time.
So we’re seeing this concurrency rising exponentially. We get more concurrency over time, we get more power, we have more powerful things we can build.
So how do you actually build an application that can handle this concurrency more efficiently? We want to be able to go from zero users to millions of users and have a compute model that works all along the way. It needs to be easy for us but also needs to be efficient.
Getting back to the idea of builders needing something that’s customized for where they are right now, I’m introducing the idea of low concurrency applications versus high concurrency applications.
I see a low concurrency application as something where it’s a niche product right now. Maybe it hasn’t quite reached broad market fit yet, so it has a few users that are interacting with it throughout the day but it hasn’t quite yet reached viral adoption. The most important thing for this application is ease of development and low baseline operational costs. If I only have a few users using the system I’m probably not making very much money off of it. Therefore, I don’t want to pay a lot of money to run the system for those users. I need to minimize the cost there.
Meanwhile, on the other side we have high concurrency applications. These applications have no shortage of users. Maybe they’re viral. They’ve got millions of users hitting the system and what they need at that point is the most efficient compute possible. They need bulk compute at the cheapest sustained usage rates. You start to have a little bit more leeway, a little bit more margin in the system to operate more complex systems and you can start to run at a higher baseline cost. But you don’t want cost to explode and go exponential as your users go exponential. You want to minimize the growth of cost as your users grow.
There’s three services I’m going to talk about for serverless containers: AWS Lambda, AWS App Runner, and AWS Fargate. And these are serverless technologies that will help you grow grow from a low concurrency application to a high concurrency application.
So starting with AWS Lambda: think of AWS Lambda as a containerized event handling function in the cloud and this function will look something like this.
This is a simple “hello world”. Obviously not very sophisticated but imagine if you want to execute this “hello world” on demand for many, many clients. There’s different ways to run this function.
One way might be with events. I can call the AWS Lambda API directly, or I can hook up Amazon EventBridge. We saw several several announcements happening with Amazon EventBridge today. I can tie those events in, so that way a handler function such as this one executes inside of AWS Lambda whenever an event happens.
But for web workloads in particular, this is where things get even more interesting and there’s a lot more concurrency. I want to be able to feed web requests to this handler function. So I could do that with Application Load Balancer. I could do that with an Amazon API Gateway, and even I can have a Lambda function URL. These are all different ways of getting a web request off the Internet and into my handler function.
Now, the interesting thing is what happens when the handler function runs. What Lambda does is it packages up the handler function inside of a micro VM. It spins up the micro VM and puts the handler function inside that micro VM to run your code in response to that event or that request. These micro VMs are strongly isolated from each other, and they’re only ever doing one thing at a time. Let me explain how that works.
Here’s the lifecycle of a lambda function instance: starting from what we call “cold start”. Cold start is when nothing is happening yet in the system. The code hasn’t ever been run, an event hasn’t happened, or a web request hasn’t happened yet. So there are no micro VMs at the time that a request arrives or an event happens.
Lambda spins up a micro VM, downloads your code into it, unzips it, and then begins initializing your code. So initialization of code is as it runs the file from the top down. Maybe you need to connect to a database, download some information to get started with, or something along those lines. This happens outside of your handler, and then from that point forward, Lambda can begin running the handler function multiple times in response to events or requests that happen.
The pricing model for this is that you pay per millisecond for the time that is spent in initialization code and the time that is spent invoking the function code. The reason why this is particularly important for low concurrency applications is that Lambda optimizes down to zero cost when there’s no work to do.
Here we see a workload where you pay per millisecond for initialization and invocation. Then there’s a second where there’s no request coming in. There’s nothing for the system to do. There’s actually no charge there. Then another request happens. We pay per millisecond, and then no requests are arriving for another 500 milliseconds: there’s no charge. So Lambda is micro optimizing down to only charging you during the milliseconds where you actually have work to be done.
But what happens when you start to get more traffic, you start to get that product market fit, and now you have multiple users using the system at the same time and multiple concurrent requests arriving? Well, here’s where Lambda has to start to spin up multiple function instances, because each of these function instances is only ever working on a single thing at a time. We see one request arrived: there’s a cold start, and it spins up a function. If a second arrives while a request is being processed, it has to spin up a second function instance in parallel. And likewise with three concurrent requests, that’s going to require three function instances.
Lambda still attempts to optimize pricing. In this case, there’s enough traffic to keep two function instances busy with back to back requests, but the third one doesn’t actually have enough requests arriving to keep it busy. So we see that the cost cost goes up and down between 3x function instances and 2x function instances.
But here’s where things get interesting. You establish that product market fit. Lambda has helped you build out product features that have become very popular, and you start to get a lot of users. All these users are talking to your Lambda function and the number of function instances are starting to stack up. Now it’s time to start thinking about other alternative models for running your code.
And to explain this, I want to do a little deep dive into what concurrency actually means and what’s happening during the lifecycle of the request.
This is a very common request that you will see time and time again in applications. It’s a simple user sign up request. It takes about 120 milliseconds to process from beginning to end and you’ll see it’s broken up into chunks here.
- The first chunk is an input payload arrives. It takes about one millisecond for the processor to validate it.
- Then the application needs to insert some data into the database. So it talks to the database over the network and it’s waiting ten milliseconds for the database to persist that data onto a disk.
- Then a response comes back, your code handles that response and maybe it makes another call to a downstream service, like an email sending service or something along those lines. Now this service takes about 100 milliseconds to finish sending the welcome email or verify your email address email.
- And then finally control returns back to your application again and the application spends eight milliseconds generating a response to send back to the client.
So 120 milliseconds, but if I look at it closely, I’ll realize that out of that 120 milliseconds, only ten milliseconds was actually spent with the processor running my code. The other 110 milliseconds was actually just spent doing nothing but waiting.
And this is very common in I/O heavy workloads. The processor, for a single transaction, will spend the vast majority of its time waiting.
As I start to look at that model of many function instances running it independently and only working on one thing at a time, I see a lot of time being spent on waiting and very little time actually being spent on the CPU doing things. But I’m paying for all those milliseconds. So what’s the alternative?
Well, every modern application language has the concept of an event loop. The event loop is a way to allow a single process to work on multiple concurrent requests at a time. Think of each second as having a thousand milliseconds of time in which to do work. And if I split my code up into small chunks of one millisecond, one millisecond, eight milliseconds, then I can schedule those chunks of code into every millisecond of those 1000 milliseconds. Whenever the CPU would otherwise be just waiting, doing nothing, I can actually grab another piece of code to do and fill that time.
Here’s how that works. One request arrives, and we start validating that payload for one millisecond. Now, while we’re waiting on the database, rather than doing nothing for ten milliseconds, we can actually grab another request off the Internet and start processing the other request by validating another input. Here you can see that these different transactions, they stack up, and your code starts working on them in parallel with each other and actually processing multiple requests at the same time in the same process.
I particularly like Node.js, my favorite runtime language. So I’ll show an example in Node.js of how easy it is to write this. Here I have a function, and I just add the async
keyword to it. Anywhere that my code would be waiting on network I/O, I can just add the await
keyword. What this does is it tells Node.js: “this is a point where I’m waiting, so you can do other things while I wait”.
Here’s what happens: Node.js splits that function up into three blocks separated by await
’s.
- The first block of validation takes one millisecond.
- The
await
takes ten milliseconds. - The second block takes one millisecond.
- The
await
takes 100 milliseconds. - Then the final block takes eight milliseconds.
But if you add that up, it only adds up to ten milliseconds. And that means that in every second of time, I can actually run this function 100 times per second and stack up 100 concurrent requests.
Now, this sounds magical, and it definitely is, but it does have some downsides. I don’t want to make it sound like this is the perfect solution to everything. The main downside is what happens when you have too much concurrency.
I said that that function could handle 100 requests per second. What happens if my clients start sending 110 requests per second? Well, what’s going to happen is that the work to do is going to start queuing up in memory, and it’s going to take longer and longer for the CPU to get around to doing that work as the queue grows in length.
Here is what you see: the memory goes up, response time starts going up as the CPU utilization reaches 100%. Eventually what’s going to happen is requests are going to start timing out. Everybody’s going to be unhappy. Clients are going to be like, “why is the service down?”
So what’s the solution here?
Well, similar to how Lambda works, we need to start running multiple copies of the application in parallel with each other, each with their own event loop and load balancing across the event loops. Ideally, these event loops are on different CPU cores or even different cores of a different machine. And each of those event loops is going to be able to process, in this case, 100 requests per second with that function that I showed earlier.
So this is a great transition to AWS App Runner, because that’s exactly how AWS App Runner is designed to function. With AWS App Runner, you set up your application and then you can tell App Runner how many requests at a time to feed to your application up to a limit that you specify.
AWS App Runner handles everything in the stack between your client code and your back end server code. That includes load balancing and ingress: getting traffic off the Internet to your application. That includes the scaling aspect, that includes the micro VM similar to Lambda.
And then you provide the application container that’s implemented with an event loop, and you tell App Runner: “I would like to be able to serve a hundred requests at a time to my app runner container”.
Here’s how that stack builds out. I have my client side code and AWS App Runner provides an automatic endpoint for my service. All I have to do is have my client send requests to that endpoint.
The end point behind the scenes is powered by an Envoy proxy load balancer, managed by AWS App Runner. Inside of that Envoy Proxy load balancer, there are limits set up according to the concurrent request limit that I set of 100 requests. The queue keeps track of how many requests are in flight to my container at any given time. If I start to reach the point where I’m about to hit that limit of 100, App Runner goes ahead and preemptively launches another copy of the application container to distribute requests across.
Now I can reach up to 200 requests at a time, because each of these application containers is being fed up to 100 requests at a time. I can set limits, so I can say, “Don’t go over two application containers”. If that happens and I start to receive, for example, 300 requests per second, 300 requests at a time, then App Runner can start to shed traffic and say, “I’m going to return a 429 Too Many Requests
error to clients”, to prevent it from impacting and causing the denial of service attack or high latency and response time for the existing clients that are connected to this endpoint.
Here’s how that model works. As you scale out, with traffic growing over time, I want to call out one important caveat, too. Lambda has cold starts. App Runner also has cold starts. It takes a certain amount of time to set up that container and get your application started. And the longer your application takes to start up, the less reactive App Runner is going to feel to traffic spikes.
Here we see that second cold start that happened as traffic grew, took a little bit long, and as a result, there was a scattering of 429 Too Many Requests
status code errors that were returned to clients. So it’s very important to make sure that you allow App Runner to scale out to an appropriate number of containers and that your application starts up as quickly as possible.
But once you get those two things working well together, you’ll find the App Runner able to launch containers to add bulk amounts of capacity at a time. Like in this case, 100 concurrent requests at a time is being added to the overall amount of capacity that this application is able to handle.
I want to talk about the pricing model. Similar to Lambda, App Runner attempts to optimize to reduce costs when you don’t have traffic. It does not optimize all the way down to zero, though. App Runner will charge for the memory of your application at all times. However, it’s going to try to charge for CPU only when you have active requests arriving.
Each time that an App Runner service activates by receiving a request, it’s going to stay active for at least 1 minute. The difference between Lambda and App Runner, however, is that once that process activates, you can serve any number of requests up to the limit that you specify, and you’re going to pay the exact same price. Whether I’m serving one request per second or 100 requests per second, I’m going to be charged the exact same amount based on the dimensions of CPU and memory. So you start to get a lower per request cost at higher traffic.
But the caveat here is that with that minimum time of 1 minute being active, if I was to receive, for example, a request every 10 seconds, it would actually keep this container active 100% of the time. It would never be able to dial back to charging only for memory.
The ideal use case for an App Runner service is a business style application that perhaps is busy during the day, during daylight hours, while people are in the office. Then everybody goes home at five. Nobody’s really using the front end application for the office. And as a result, App Runner can dial back to only charging for memory because there’s no requests arriving overnight. You’ll find that this does provide a lot of optimization for some business use cases because memory is the extremely cheap dimension compared to CPU. You can add gigabytes of memory and not even begin to approach the cost of adding cores of CPU.
So this works together with scaling. As traffic rises over time, we see that it starts out as charging for one application instance at the cost of CPU plus memory. Then traffic continues to rise and we have to scale out to two. So we are being charged for 2x CPU and memory. But then when traffic stops, App Runner dials back to having two container instances that are only charging for memory and then only one that’s only charging for memory. You can start to imagine how App Runner will scale up and down and how costs scale.
This leads to the third model, AWS Fargate. So with AWS Fargate, we once again have a serverless container similar to Lambda, similar to App Runner, but there’s a lot more pieces of the puzzle that are up to you to handle, and this can be both a blessing and a curse. It can be a little bit hard to configure these things at times, but on the other hand, you have the ability to optimize things exactly how your application functions and potentially reach even lower price for handling large amounts of traffic.
I want to talk about first what AWS Fargate does handle for you, and that is patches to the underlying host and infrastructure. So AWS Fargate is providing micro VM’s on demand for your application. You don’t have to think about those. For example, we had different SSL patches that happened in the past. And all of those were handled by AWS. All I had to do was keep my application running in AWS Fargate, and the underlying hosts and the host operating system are all patched.
I do have to manage though, the runtime inside that container, so that’s a little bit different. Lambda will patch the runtime inside the container, but I have to patch the runtime inside of a container on Fargate.
Load balancing and ingress. This is another big one. If I’m running an internet connected workload and I’m receiving traffic from the Internet with AWS Fargate, I have to choose what my ingress and load balancing to use, and pay for that separately, whereas App Runner bakes the price of the ingress into the App Runner service.
And then last but not least, the scaling. So, whereas App Runner and Lambda have a built in scaling model, Fargate does not have a built in scaling model. You have to choose how many Fargate containers you would like to run at any given time and make sure that you’re running enough Fargate containers to handle the traffic volume that you are actually receiving.
So how do we do that? This is where an orchestrator comes in. An orchestrator is a piece of code which is able to take your intent for how you would like your application to run and try to obey your intent to make sure your application runs properly.
Here is an example. Developers can tell the orchestrator, “I’d like to run ten copies of the app. I’d like to register those copies of the app into this load balancer that I provisioned. And I would like you to gather the metrics and stats from my running containers. And if the traffic goes so high that CPU goes over 80%, then I’d like you to start scaling up and adding more containers.”
You have a choice as to which orchestrator you’d like to use. AWS Fargate allows you to use either Elastic Container Service or Elastic Kubernetes Service as the orchestration API for handling these micro VM containers inside of AWS.
ECS or Elastic Container Service is a fully AWS managed API and I think it fits best with the serverless model. It is a serverless API. You don’t pay anything for it, you use it on demand and it is orchestrating AWS Fargate on your behalf so that you only pay for the AWS Fargate tasks.
On the other hand, AWS Elastic Kubernetes service is an open source deployment. So AWS is going to deploy some physical pieces of hardware that are going to be running this open source project on your behalf. You do have to pay for the cost of running that hardware.
In addition to the AWS Fargate cost, another choice that you have with AWS Fargate is how you want to receive the traffic. Application Load Balancer is best for if you have a HTTP, gRPC, or WebSocket style application where you want layer seven, or application aware load balancing to your back end.
Network Load Balancer is best if you want low level raw TCP or UDP, packet level and connection level load balancing. Network Load Balancer is a bit cheaper, but you get a lot more features, a lot more power, and a lot smoother of load balancing with an Application Load Balancer.
Amazon API Gateway is a serverless ingress, so the idea with that one is you only pay when a request arrives. You pay per request that hits the Amazon API Gateway. The disadvantage is Amazon API Gateway can start to get a little expensive as you get many, many requests, compared to Application Load Balancer and Network Load Balancer. ALB and NLB, they have a higher baseline cost, so if you have low traffic, it’s going to look like API Gateway is cheaper for you. However, as you get really high traffic, you’ll start to notice API Gateway costs adding up compared to an ALB or NLB, which can handle large bulk amounts of traffic at a fairly low rate.
I will talk a little bit about the scaling and scaling options do you have with Amazon ECS. The scaling is integrated into AWS Auto Scaling and Amazon Cloud Watch. You have options:
-
Step scaling is for if you want to define extremely custom scaling rules, like at this particular CPU threshold, add this many tasks and this many containers.
-
Target tracking is if you want AWS to figure things out on your behalf. You say: “I’d like you to try to keep the CPU utilization at around 80% and figure out how to do that.” AWS is going to play with your task number and increase it and decrease it until it finds the right balance.
-
Scheduled scaling is fantastic. If you have known scheduling events, for example, you know that at this time there’s going to be a lot of people that are going to start using your service, or at this time of day, traffic increases or decreases.
And you can scale across any type of metric. There are built in metrics like CPU, memory and network I/O, but you can also hook in your own custom metrics, or metrics that are application specific. For example, for a worker that’s pulling work from an SQS queue, you might be interested in knowing how many messages are in the queue and scale according to that.
The fundamental difference with AWS Fargate from the Lambda model or the App Runner model is that AWS Fargate charges based on time, not activity. AWS Fargate does not actually care whether you have any traffic at all to the container. So if I have zero traffic, I’m going to be paying the same rate as if I’m serving 200 or 300 requests per second to that task.
You’ll start to realize though, that as you max out your Fargate task, you keep them busy with lots of work and lots of requests, that Fargate does, however, give you the lowest per request cost compared to a Lambda style, which at that same level of traffic would add up to a lot more cost.
There are also options you can use to reduce the Fargate price even more, such as adjusting the CPU and memory dimension down to the lowest size. So traditionally, if you’re spinning up a VM, there’s discrete sizes and you can only scale your VM down so far before you can’t get any smaller VM that has a lower cost. With AWS Fargate, you can always go all the way down to 256 CPU, which is a quarter of a CPU and 512 megabytes of memory. So you’re paying for a very small slice of compute capacity with a low cost.
AWS Fargate is also part of the Compute Savings plan. If you know you’re going to be running this application for a long time, maybe even years, you can pre-commit to paying the cost of that application and receive a flat percentage discount on the cost.
And the other way that I’ve been recommending to folks is to consider Graviton and ARM based tasks. The reason why is when you spin those up, you’ll notice you’ll get a much more powerful processor at a lower cost per minute and per second on AWS Fargate.
So now we get to the question, and this is where we start to balance the efficiency versus ease, “How do I know which serverless option is right for my application?” The thing I would encourage everyone to do is think not just “is it right for my application now?”, but also “will it be right six months from now or a year from now?” and find that right balance between the ease and efficiency. Let me look back at some of these dimension so we can compare them side by side.
Pricing Model:
- With AWS Lambda the pricing model is pay per millisecond per invocation based on the memory size of the function and every single one of your concurrent invocations is going to stack up and be charged separately.
- With AWS App Runner the pricing is based on second. Once again. But it’s based on the CPU size and the memory size. You have two dimensions there. Instead of just memory, you have CPU and memory. And App Runner is able to optimize down to only charging for memory when you have no traffic. But then when you do receive traffic, it’s going to charge a flat rate for the CPU, no matter how much traffic is arriving to that container.
- AWS Fargate has a constant price based on CPU and memory, whether you’re receiving any traffic or not.
The pricing resolution is important:
- With AWS Lambda, it can scale to zero between each request, even at the millisecond resolution. So if you have one request arrived and then a gap of seconds or milliseconds before the next request arrives, AWS Lambda is able to optimize all the way down to zero in between those gaps.
- With AWS App Runner, every time the application instance activates, it activates for a minimum of 1 minute and the duration is rounded up to the nearest second. So you’ll see a little bit less resolution there. But if your traffic has a pattern where it goes up for a period of time and then it goes down and there’s very little traffic, App Runner is going to be able to fit that resolution.
- Then for AWS Fargate the pricing is calculated per second with a 1 minute minimum, once again, similar to App Runner. But it’s going to charge from the time that the task starts until the time that the task stops, and it’s up to you to optimize the starts and stops to make sure that you’re not running a container that is not actually doing anything.
Scaling model:
- Lambda has built in scaling. It’s going to only ever serve a single invoke at a time from a function instance, but it’s going to increase the number of function instances on the fly extremely quickly, extremely reliably, and be able to handle spikes very, very quickly.
- App Runner also manages a number of container instances for you. It can be a little bit slower to respond. I would say on average, like I’ve seen with Lambda, cold starts as low as ten milliseconds. With App Runner it’s not uncommon to have a cold start of about 30 seconds. The difference though, is that when you add an App Runner container you’re adding a pool of capacity that’s much larger, adding potentially 100 concurrent requests to your available capacity pool. So App Runner can be slower to respond, but when it does respond, it adds a larger chunk of capacity. And this still allows it to keep ahead of traffic as long as the traffic doesn’t spike from zero to very high instantly, as long as there’s some kind of curve to it, App Runner can respond in time.
- AWS Fargate has no built in scaling whatsoever, but it can handle really high concurrency. You have to define your own custom scaling rules and decide how many containers are appropriate to run at any given time and make sure you’re not running more than you need and paying more than you need. But there’s help. You can use an orchestrator, you can use AWS Auto Scaling which provide some tools out of the box, as well as custom logic that you can implement yourself.
Web Traffic and Ingress:
- With AWS lambda, you have a choice. There is a built in function URL that doesn’t cost anything. Additionally, there is API gateway, which is a serverless model that’s going to pay per request that arrives, there’s no cost when there’s no request. Or you can even use an Application Load Balancer and hook that up to AWS lambda. Application Load Balancer, though, obviously has a constant hourly charge whether you’re receiving traffic or not.
- With AWS App Runner, all the ingress is built in. You don’t pay anything additional for ingress because that Envoy proxy load balancer is being managed by AWS App Runner behind the scenes for you.
- With AWS Fargate, there’s no included option. So whereas Lambda has function URL and App Runner has a built-in Envoy proxy Fargate does not come with a built in ingress, you have to provide your own ingress. And it’s up to you whether you want to use a serverless model of, for example, an API gateway, or whether you want to use a serverful model like an Application Load Balancer.
And the choice there really comes down to traffic level. If I have low traffic, maybe API gateway makes sense for me. If I have really high amounts of concurrent traffic, maybe Application Load Balancer makes sense.
Here’s the map of how I think about sweet spots for your application, when you’re looking at that ease versus efficiency. If we’re looking at concurrency, the dimensions are: concurrency intensity and concurrency stability.
Intensity is how much concurrency are you dealing with? Are you getting a scattering of requests, maybe one request at a time or a few requests at a time? Are you receiving large amounts of traffic hundreds or even thousands of requests at any given time?
The other dimension is concurrency stability. How predictable is your traffic? For example, I worked in the past at a social media company where there was a very predictable pattern. In the morning, traffic would start to rise. At lunchtime, as people get off work, the traffic would rise and fall back down. As people get off work in the evening, around five, through each time zone, the traffic would rise and rise and rise throughout the evening until finally people went to bed. So every day I could rely on that particular pattern, and if I didn’t see that pattern, I knew something was terribly wrong with the application.
So that’s an example of a very stable, predictable concurrency. Whereas imagine like something like a ticketing solution and Taylor Swift is about to have her concert and a million people are trying to buy Taylor Swift tickets. That’s a very spiky workload: out of the blue I could have millions of people hitting the backend and trying to send requests.
AWS Lambda, it excels at workloads that are extremely spiky and it excels at workloads where there are few requests at a time or large gaps in between requests because of that pricing model, because of that concurrency model, and because of that scaling model.
On the other hand, you’ll see AWS Fargate at the far end of the spectrum. It excels when you have constant high levels of traffic, hundreds or thousands of requests at a time, and you have a fairly stable and predictable pattern because it’s easier to build auto scaling if you can predict what the scale is going to be like.
AWS App Runner sits somewhere in the middle. As I mentioned before, it’s ideal for a back office application which is used during the day. You have stable, predictable traffic during the day, but then when people go home at night, the traffic sort of dwindles down to nothing and now App Runner can scale down to only costing for memory.
So this is how I personally think about it. The other dimension to think about is what is included out of the box. Obviously, building your own scaling rules for AWS Fargate can be a little bit challenging, so that doesn’t really hit the ease dimension. But by the time you have hundreds or thousand of requests at a time, it’s okay to spend a little bit of time with development effort figuring out scaling because it’s now worth it to you. Whereas if you’re just building your first greenfield project and you’re focusing on building business features first and foremost, maybe it doesn’t make sense to spend as much time thinking about scaling, thinking about ingress. Maybe I just want to use the easier approach of AWS Lambda.
There’s one more dimension that I want to talk about. This one is important to consider if you have an application that has regulatory requirements or high security requirements. AWS Lambda is the only option that has per request isolation. So what that means is one request arrives and another request arrives: those are requests are each being processed on their own independent processor which is dedicated to handling that request and that request alone.
This can be important if your workload is dramatically different from request to request. For example, maybe one request consumes a lot of CPU and other requests only consumes a little bit of CPU. Or perhaps the requests are untrustworthy. An example that I built before in the past was I was asked to build a solution that would take a screenshot of an arbitrary user submitted URL. Now, I would say that’s scary because they could put any URL on the Internet. I don’t know what that website is that I’m screenshotting. It could have malicious code in there that’s trying to hack out of the sandbox and break into the system. So I definitely ran that in Lambda because it’s perfect for it. Each particular screenshot is happening in its own sandbox micro VM, independent of anything else that’s happening in the system.
Now, the downside of that per request isolation though, is you can’t take advantage of certain optimizations, like in-memory caches. Like with Lambda, if I have 100 concurrent requests, each of those has 100 different function instances, so there’s 100 different in-memory caches. An in-memory cache is not going to really perform the same as it will on App Runner or AWS Fargate, where one process is handling 100 concurrent requests and is able to take advantage of cache or recently fetched results that could be stored in memory.
So definitely consider that. Once again, you’ll notice that App Runner and Fargate are a little bit better for a super high volume of requests because of that.
But on that ease and efficiency spectrum here’s where I would place these products:
- AWS Fargate is very configurable. It gives you a lot of control. The downside is it’s a little bit more complex. It’s not going to be as easy to set things up with AWS Fargate because you have to set up rules and configuration for everything that you want to happen.
- AWS Lambda is on the other end. It’s very opinionated. You don’t have to set up a lot of configuration. It’s fully managed by AWS, and it’s a little bit simpler and easier to get started with. Downside is you may not be able to optimize costs down to as low as you would be able to at large amounts of traffic on AWS Fargate or App Runner.
- App Runner is a little bit in the middle. I would put it a little bit closer to Lambda than AWS Fargate in terms of things that’s trying to take off your plate for you, like scaling and ingress. But you can still configure more of those dimensions and settings as you see fit.
So thank you very much for paying attention to this presentation. I’d be happy to take questions if anybody has some questions I can help answer.
You can reach out me with questions on Twitter at @nathankpeck